Nuclearn Platform v1.4.0 is by far our biggest release yet! This release brings a lot of functionality we have been excited about for a long time to our customers. While the detailed release notes are quite extensive, there are 4 major enhancements that will interest most customers:
- CAP Screening Automation & Workflow Automation General Availability
- Improvements to Dataset and Dataset Analytics
- Kubernetes Support
- Azure AD Authentication Support
CAP Screening Automation & Workflow Automation General Availability
Nuclearn’s Workflow Automation features have been in preview since Q4 2021, and are core to enabling our CAP Screening Automation products. With Nuclearn v1.4.0, these features are now generally available for our customers using our CAP Screening Automation module! This release exposed the capabilities to build automation templates and configurations via the web interface, making it very easy to set up new CAP Screening Automations.
This release ties the Automation workflows much more closely in with our existing Dataset and Model functionality, making it even easier to deploy, maintain, monitor, and audit CAP Screening Automations. Additionally, the functionality added in this release makes it very easy to apply Nuclearn’s Workflow Automation to other processes beyond CAP Screening!
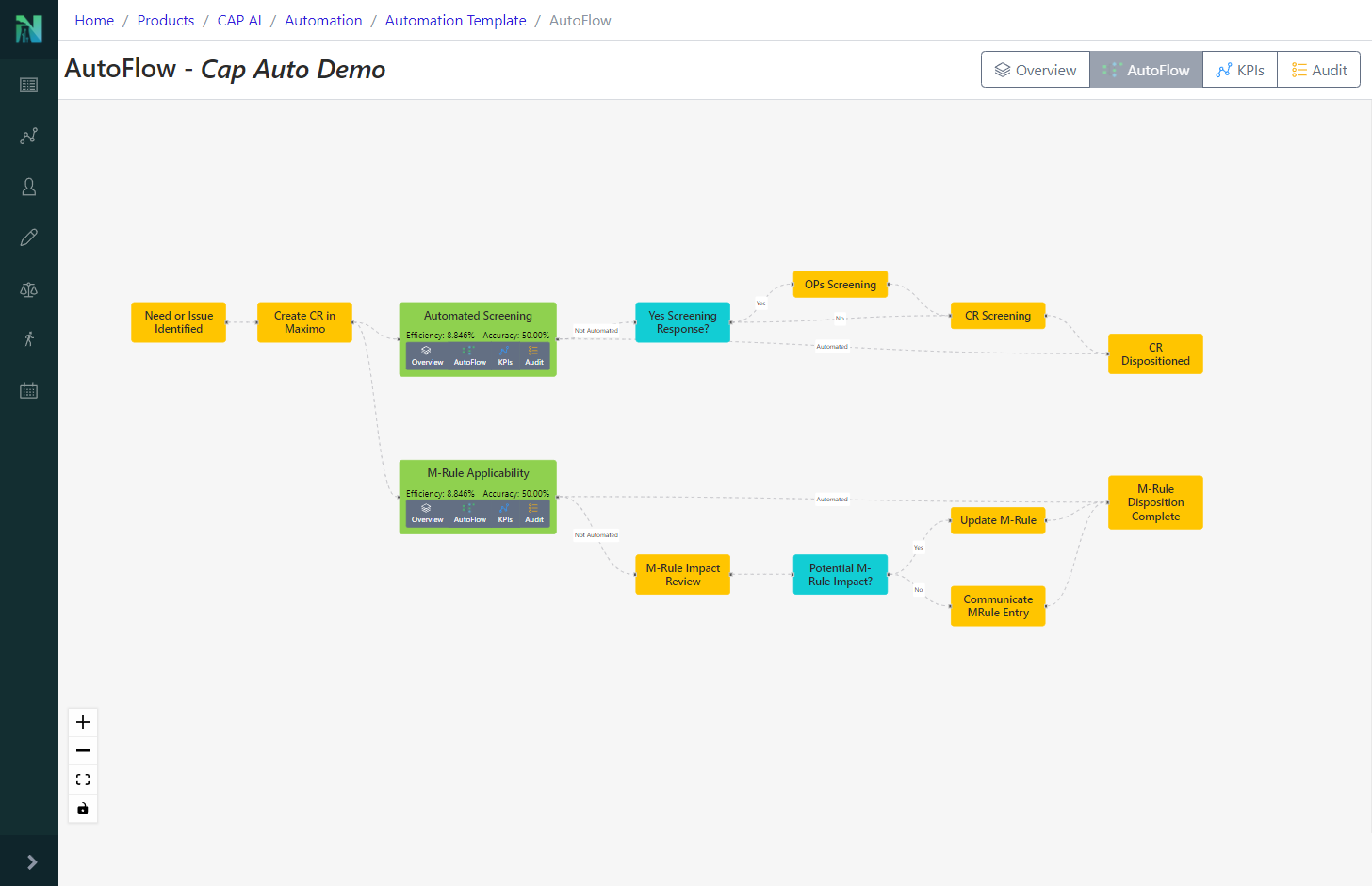
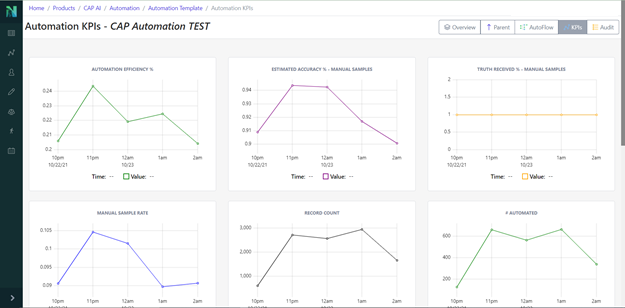
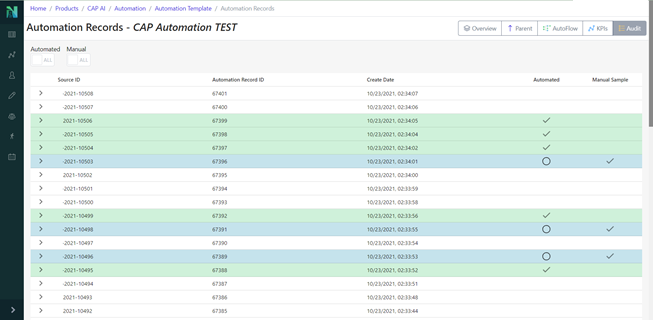
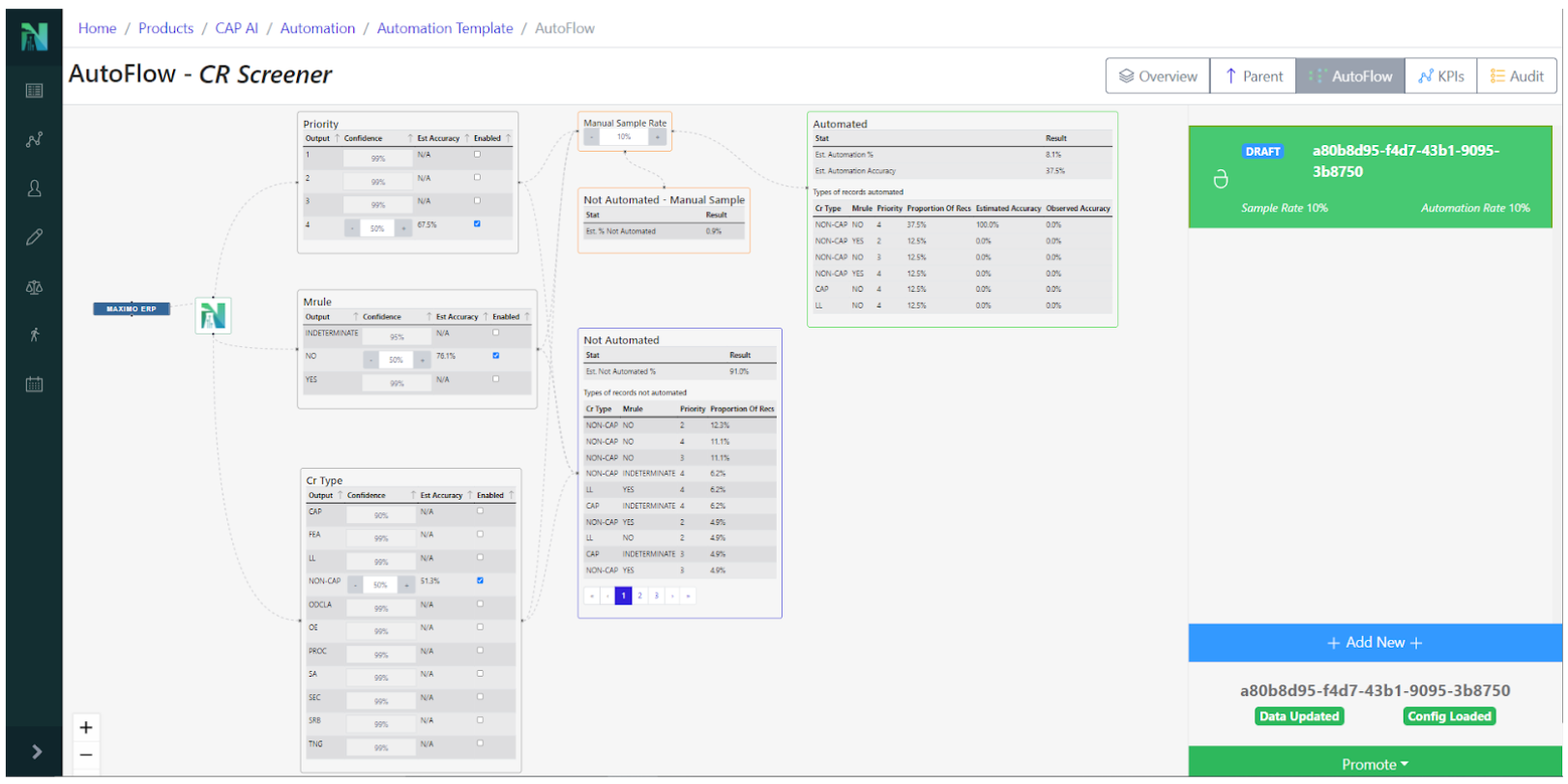
Improvements to Dataset and Dataset Analytics
v1.4.0 brings many new user experiences and stability enhancements to the Dataset and Dataset Analytics feature added in 1.3. These include a far more intuitive user interface, progress bars for monitoring the status of long-running scoring jobs, more flexible analytic options, and numerous bug fixes. These enhancements should make using Datasets for INPO Evaluation Readiness Assessments or Continuous Monitoring even easier!
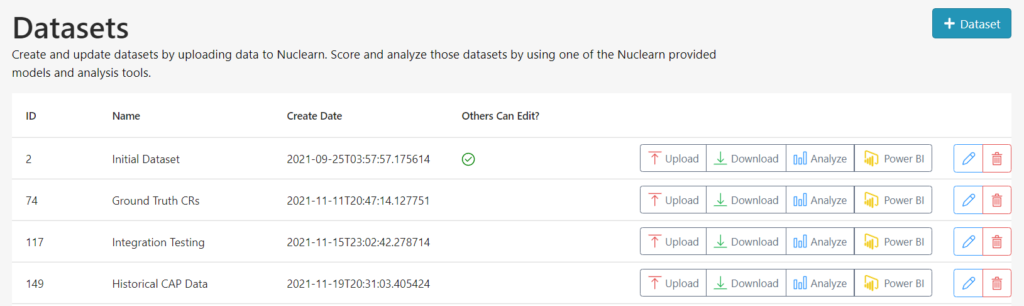
Kubernetes Support
With the release of v1.4.0, Nuclearn is now supported on Kubernetes. As many enterprises move their containerized applications to Kubernetes, this is an important addition to the platform. Nuclearn Platform releases now included a Helm Chart for the entire platform and detailed instructions for configuring Nuclearn for Kubernetes. We have found that our deployments are actually easier to configure and install on Kubernetes, in addition to the horizontal scalability and fault tolerance a deployment on Kubernetes provides.
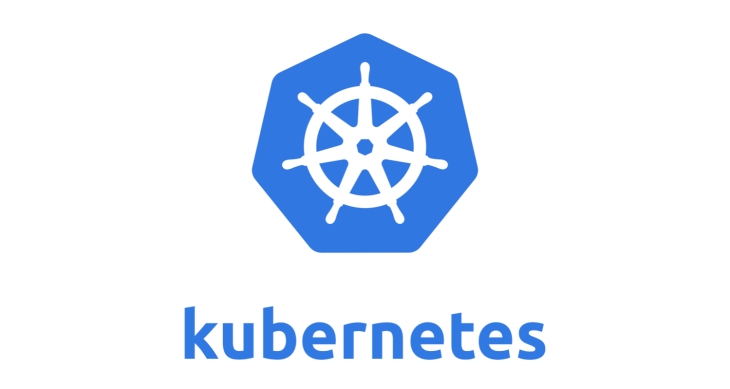
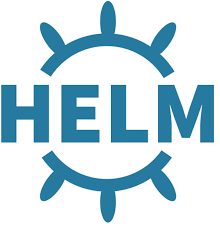
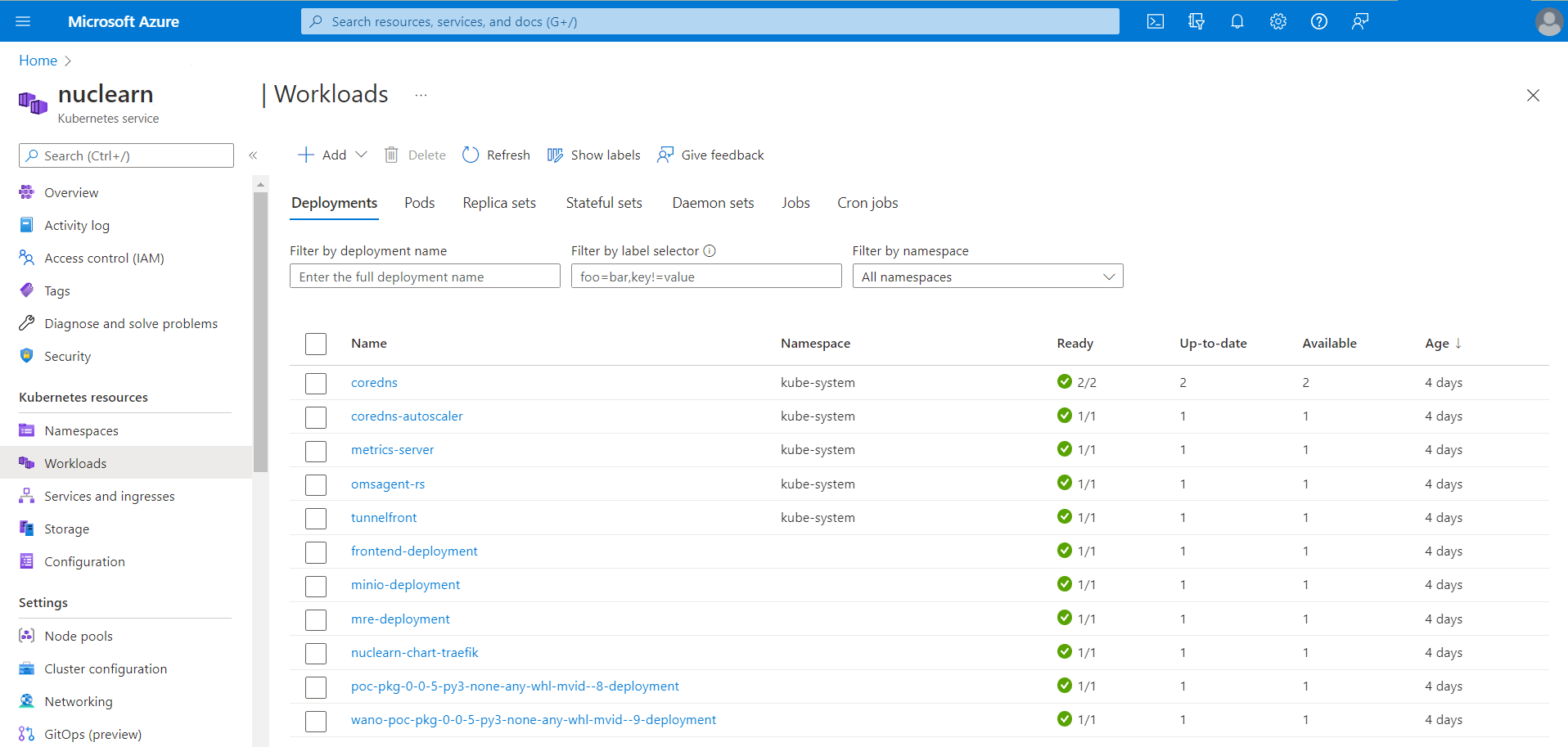
Azure AD Authentication Support
In addition to Active Directory authentication via LDAPS and native application authentication, Nuclearn v1.4.0 includes top-level support for Azure Active Directory (Azure AD) authentication. Customers leveraging Azure AD authentication within Nuclearn are able to SSO into the Nuclearn platform, and easily configure group permissions with Azure AD.
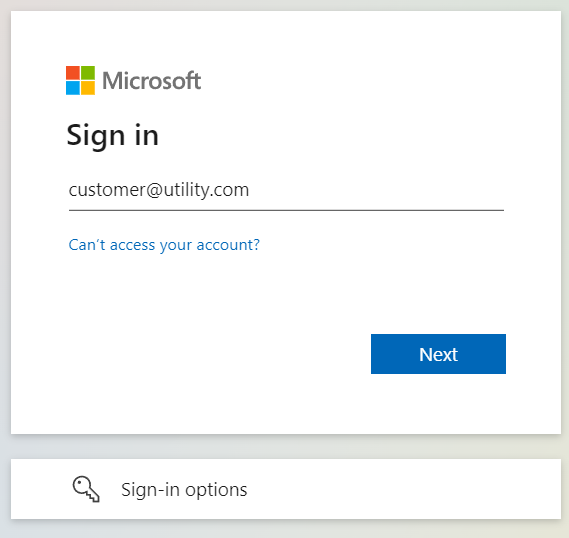
Beyond the most notable items already listed, there are even more miscellaneous enhancements and bug fixes. Additional details can be found in the detailed release notes below.
Nuclearn Platform Release Detailed Notes
v1.4.0
Highlights
- Workflow Automation General Availability
- Improvements to Dataset and Dataset Analytics
- Support for Kubernetes
- AzureAD authentication support
Updated Web Frontend To v1.2
- Workflow Automation General Availability
- Forms for creating, editing and deleting automation templates from scratch
- Ability to view parent and child automation templates
- Automation template overview page
- Improvements to autoflow configuration page
- Ability to kick off automation config test runs directly from the frontend
- Several fixes to audit details page for correctness and performance
- New KPI page for each automation template
- Automation template can now either render automation configuration for a model or be a “parent” automation with children automations, and pass global parameters down to children
- Improvements to datasets and dataset analytics
- Redesigned dataset UI buttons to be more intuitive
- Adding a progress bar during dataset scoring
- Added ability to select whether to include first and last time periods in dataset analytics
- Added “week” as an option for time grouping in dataset analytics
- Added ability to directly download raw predictions from analytics page
- Added ability to download an entire dataset as a csv file
- Improved error messages for dataset uploads
- Major enhancements to model and model version management
- Changed model details routes to no longer be product specific
- Standardized and improved model deployment buttons
- Added new forms for creating models from scratch
- Added new forms for creating new model versions and uploaing supporting whl files
- Model admin page now uses collapsing cards for models and model versions to make UI easier to navigate
- Most API calls related to models migrated from axios to ReactQuery, which will improve performance and enable better UI in the future
- Most model react components migrated from legacy classes to react-hooks
- “Predict Now” can now support models that require more than one input field
- Fixed bug where UI would not render if a model did not have an associated model version
- Misc
- New login page for supporting AzureAD authentication
- Fixed bug where users had to login twice after their session times out
- Minor UI fixes to fit pages without scrolling more often
- Improved loading icons/UI in many place across application
Update Model Engine to v1.3
- Workflow Automation General Availability
- Many additional routes for supporting Automation Templates, Configs, Audit, and KPIs on the frontend
- Added ability to specify parent/child automation config templates
- Added ability to provide configuration data for an automation config template
- Refactored “Test Runs” to be generated from an automation template, dataset, and model version instead of just a model version
- Automation configuration templates can now be tied to a “ground truth” dataset
- Accuracy is now calculated and saved on the automation data record rather than calculating on the fly
- Added unique constraint on AutomationConfigTemplate names
- No max name length limit for automation configuration templates
- Soft deletes for automation configuration templates
- Removed hardcoded product ids and automation configuration template ids from routes and operations
- Updated permissions and roles across all automation config routes
- Updated testdata routes to still return model labels if no test runs have been completed
- Dataset & Analytics Improvements
- Added “week” as a valid option for dataset stat timeslices
- A central dataset scoring queue is maintained so that multiple requests to score a dataset do not conflict
- Added scoring progress route to check scoring progress
- Improvements to csv upload validation, including checking for null UIDs, verifying encoding is either UTF-8 or ascii, and other misc improvements
- Added route for downloading dataset data as a csv file
- Added route for retrieving scored model predictions as a csv file
- Added support to dataset stats for including/excluding first and last time periods
- Model Deployment & Orchestration Overhaul
- Support for multiple model backends
- DockerOrchestrator and KubeOrchestrator added as supported model backends
- Configuration for multiple backends provided via mre-config “ModelOrchestration” entry
- Disable undeploying models on startup by setting ModelOrchestration -> undeploy_model_on_startup_failure = false
- Orchestrators are now mostly “stateless”, and query backends to retrieve model status
- Major improvements to model binary handling
- Added routes for creating and deleting model binaries
- Better support for uploading new model binaries and tying to model versions
- Significant performance improvement in get_model_binary route
- Ability to provide pre-signed temporary tokens to model orchestration interfaces to download binaries from MRE rather than MRE having to push model binaries to containers directly
- Fixed bug where updating an existing model/dataset mapping would fail
- Added routes for creating new models and model versions
- Changed model deletions to be soft deletes
- Removed “basemodel” tables and references as it was no longer used after model refactors
- Better GRPC error handling
- All model inference operation now support or use field translations to map input data to required model inputs
- Support for multiple model backends
- Kubernetes support
- Support for MRE sitting behind a “/api” root path by setting the “RUNTIME_ENV” environment variable to “K8S”
- Added KubeOrchestrator model orchestration interface
- Azure AD Support
- Azure AD added as a supported authentication provider
- Users now have a “username” and a separate “user_display_name”. These are always the same except for users created via AzureAD as AzureAD does not use email addresses as a unique identifier.
- Added functions for syncing user roles with remote authentication providers
- Misc
- Created new model version entries for the Wano PO&C Labeler and PO&C Labeler
- Old model versions are undeployed by default and new model versions are deployed by default
- Existing integrations via the APIs may break if they specify v1 of the labeler models
- Configuration file location is now set via the “CONFIG_FILE” environment variable
- Added support for deprecating and removing API routes
- Deprecated routes can be forced to fail by setting the “STRICT_DEPRECTATION” environment variable to “true”
- Deprecated following routes:
- PUT /models/initial
- Removed following routes:
- DELETE /models/{model_id}/version/{version_number}/
- GET /models/{model_id}/version/{version_number}/basemodel/binary/
- PUT /models/{model_id}/version/{version_number}/binary/
- POST /models/{model_id}/version/{version_number}/testrun/
- Allow field descriptions for source data record fields to be null
- Trigger added to SourceDataRecord table to keep full version history in a SourceDataRecordHistory table
- Improved logging and error handling by logging in more places and creating more specific errors
- Switched many routes to “async” to reduce blocking operations in FastAPI
- Fixed bug preventing admins from updating user roles
- Created new model version entries for the Wano PO&C Labeler and PO&C Labeler
Update Application Object Storage to v1.1
- Updated WANO & PO&C model to 0.0.5, fixing a protocol bug where JSON serialization artifacts may be included in the input into the model